The COVID-19 pandemic has brought the world to a standstill in 2020-2022, causing widespread panic and leaving many governments struggling to contain the virus. Secondary effects of the pandemic (supply chain disruptions, economic downturn, etc.) are still felt in 2023. Even though the challenges of the pandemic are not fully resolved, behavioural data science has emerged as a valuable tool for understanding and preventing the spread of the COVID-19 virus.
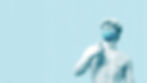
Could something as simple as a behavioural data science model, designed specifically to understand the risks and susceptibility of vulnerable people to COVID-19, help us better understand how they can embrace the "new normal" post-lockdown in a way that still mitigates the risks of the virus? In a recent study, we proposed such a model, which allowed us to save over 3,000 lives. The study explored, using behavioural data science methodology, how practical options and technological interventions, from Bluetooth wristbands to proximity sensors, can help us to achieve better outcomes for vulnerable groups alongside effective incentives to motivate people.
Behavioural data science combines insights from behavioural science with advanced computational techniques, such as machine learning and deep learning, to model, understand, and predict behaviour. In the context of the pandemic, this interdisciplinary field is being used to analyse data on factors such as social distancing, mask-wearing, and vaccination rates, with the goal of developing more effective public health interventions.
Contact-tracing, which has been used alongside other protection measures across the world to help achieve behavioural change, was supposed to keep a record of any new infection cases and anyone who has been close to them. Many contact-tracing app solutions have been developed worldwide with the view to enable uninfected and immune people (e.g., people with the so-called “hidden immunity”) to leave their homes, while people who might have been infected instructed to self-isolate. Bluetooth technology has been used previously for messaging and tracking of nearby devices using proximity detection. Apple and Google along with many health authorities have proposed software smartphone hosted apps using Bluetooth Low Energy (BLE) to automate the contact tracing process. BLE is a form of wireless communication designed especially for short-range communication suitable for situations where battery life is preferred over high data transfer speeds.
While an important part of the fight against COVID-19, a contract-tracing app is realistically not a viable option for everyone. For instance, vulnerable and older people are more likely to use older smartphones without the BLE feature. Other vulnerable groups (specifically, those who have had an organ transplant and are on immune suppressive therapy; have had a bone marrow transplant in the last 24 months; are on immune suppressive therapy for graft versus host disease; have blood cancer such as leukaemia, lymphoma or myelodysplastic syndrome; are having chemotherapy or radiotherapy; chronic kidney failure; heart disease; chronic lung disease; a non-haematological cancer; diabetes, obesity, chronic liver disease, neurological conditions such as stroke or dementia, etc.) might not see the direct benefit of downloading and using the app as they do not understand the underlying incentives behind contact tracing and how it can benefit them.
Long-established compartmental epidemiological models like the Susceptible-Infected-Removed (SIR) model and the Susceptible - Exposed - Infectious - Recovered (SEIR) model do not account for the variability encountered in the severity of the COVID-19 disease across different population groups. In a way, they are designed to provide “one-size-fits-all” solutions. Yet, under circumstances when some population groups are a lot more vulnerable to the virus than others (elderly, people with pre-existing health conditions, etc.), these groups may need extra help and more customised solutions to help them decrease or avoid the risk of being infected.
To overcome the limitation of the current models, we proposed a new model - Susceptible - Exposed - Infectious - Recovered for the vulnerable (SEIR-v) model - through which the population could be segmented into groups according to their vulnerability to coronavirus.
This segmentation enables the analysis of the epidemic’s spread when different contention measures are applied to different groups in society. These measures depend on their vulnerability to the disease. This approach saves lives. Using this novel epidemiological as well as behavioural segmentation, we proposed three possible solutions to support vulnerable people: (i) contact tracing wristband or wearable; (ii) a social distancing alert mechanism; and (iii) a wearable to monitor symptoms. The real-world pilot of these wearable solutions was conducted in Nottingham (UK) and allowed to significantly decrease the risk of contracting COVID by vulnerable groups.
This project showed how behavioural data science could help suggest effective incentive-compatible mechanisms for “smarter” adoption. Specifically, incentives may be more effective if they appeal to people’s “present bias,” which is the tendency to pursue smaller and more immediate rewards rather than bigger and more abstract goals, such as “eradicating COVID-19 in the world.” Considering that vulnerable populations generally tend to procure (health)care services more often, the function of contact tracing is likely to succeed if it is embedded into general care applications and services. For example, framing the contact tracing technology as a “digital nurse” for vulnerable groups aims to monitor (in real-time) the state of the wearer and potentially offer some desirable care features (e.g., health monitoring, automated calls for medical help, etc.). Specifically, incentives may be more effective if they appeal to people’s “present bias,” which is the tendency to pursue smaller and more immediate rewards rather than bigger and more abstract goals, such as “eradicating COVID-19 in the world.”
In circumstances when much uncertainty still exists about the characteristics of COVID-19, we show how a reduction in the exposure of vulnerable individuals can minimise the number of deaths caused by the disease (using the UK as a case study). While the effectiveness of contact tracing hinges on how many people use it (and self-isolate if they are notified that they have been in contact with someone who has the virus), it is proposed that governments could provide vulnerable individuals with a BLE wristband, capturing populations usually missed by systems relying on smartphones and an app only. Having a clear value proposition, which would go beyond the functional purpose of tracing would allow the technological solutions to succeed with the elderly population more easily, as users will see not only how their data can benefit society, but also how their data can help them receive better, more efficient, and higher quality care.
Selected References
Anderez, D., Kanjo, E., Pogrebna, G., Kaiwartya, O., Johnson, S.., Hunt, J. (2020) A Covid-19-based Modified Epidemiological Model and Technological Approaches to help Vulnerable Individuals Emerge from the Lockdown in the UK, Sensors, 20(17), 1-19.
Pogrebna, G., & Kharlamov, A. (2020). The impact of cross-cultural differences in handwashing patterns on the COVID-19 outbreak magnitude.